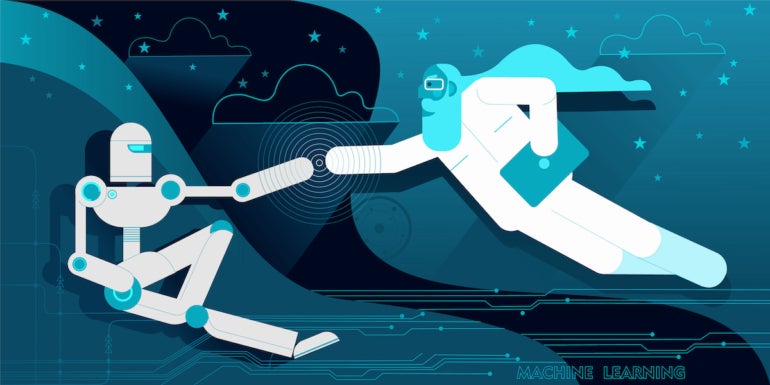
Salesforce, which last year introduced its Einstein AI framework behind its Customer 360 platform, has published what it says is the industry’s first Guidelines for Trusted Generative AI. Written by Paula Goldman, chief ethical and humane use officer, and Kathy Baxter, principal architect of ethical AI at the company, the guidelines are meant to help organizations prioritize AI-driven innovation around ethics and accuracy — including where bias leaks can spring up and how to find and cauterize them.
Baxter, who also serves as a visiting AI fellow at the National Institute of Standards and Technology, said there are several entry points for bias in machine learning models used for job screening, market research, healthcare decisions, criminal justice applications and more. However, she noted, there is no easy way to measure what constitutes a model that is “safe” or has exceeded a certain level of bias or toxicity.
NIST in January issued its Artificial Intelligence Risk Management Framework as a resource to organizations “designing, developing, deploying, or using AI systems to help manage the many risks of AI and promote trustworthy and responsible development and use of AI systems.”
Baxter said she gave feedback on the framework and participated in two of the three workshops that NIST ran to get feedback from the public and raise awareness.
“The Framework discusses what is required for trustworthy AI and the recommendations are similar to our Trusted AI Principles and Guidelines: valid and reliable, safe, accountable and transparent, explainable, privacy-enhanced, and fair. Salesforce breaks things out a bit differently but all of the same concepts are there,” she said.
SEE: Artificial Intelligence Ethics Policy (TechRepublic Premium)
How slicing and dicing data creates biased models
“We talk about AI as if it were homogenous, like a food additive that the FDA can assert is safe beneath a certain concentration, but it’s not, it is highly varied,” said Baxter, citing a 2021 paper by MIT researchers Harini Suresh and John Guttag that delineates a variety of ways data can be too narrowly used in the development of machine learning models.
Baxter said these can lead to five real-world harms.
Historical bias
Historical data, even if “perfectly measured and sampled,” can lead to harmful outcomes, noted the MIT paper. Baxter said an illustration of this would be accurate historical data showing that Black Americans have faced redlining and different standards for receiving loans.
“If you use historical data to predict the future, the AI will ‘learn’ not to give loans to Black applicants, because it will simply replicate the past,” she said.
SEE: Build your machine learning training library with this ebook bundle (TechRepublic Academy)
Representation bias
Because a data sample underrepresents some part of the population, it fails to generalize well for the subset.
Baxter noted that some vision models trained on data collected primarily from the U.S. or Western countries fall short because they miss cultural representations from other countries. Such a model might generate or find white “wedding dresses,” based on Western aesthetic ideals, rather than those of, say, South Korea or Nigeria.
“When collecting data, you must consider outliers, the diversity of the population and anomalies,” she said.
Measurement bias
The MIT paper noted that this bias results from the use of concrete measurements meant to be an approximation of an idea or concept not easily observable. Baxter noted that the COMPAS recidivism algorithm is a prime example of this: It is designed to help enforcement choose parolees based on potential for re-arrest.
“If you were to speak with the community impacted, you’d see a disproportionate bias around who is flagged as high-risk and who is given benefit of doubt,” she said. “COMPAS wasn’t predicting who is going to recommit crime, but rather who is more likely to get arrested again.”
Aggregation bias
This is a species of generalization fault in which a “one-size-fits-all” model is used for data with underlying groups or types of examples that should be considered differently, leading to a model that is not optimal for any group or one valid only for the dominant population.
Baxter noted that, while the example in the MIT paper was focused on social media analysis: “We are seeing it present in other venues where emojis and slang are used in a work setting.”
She pointed out that age, race or affinity groups tend to develop their own words and meanings of emojis: On TikTok, the chair and skull emoji came to signify that one was dying of laughter, and words like “yas” and “slay” come to carry specific meanings within certain groups.
“If you attempt to analyze or summarize sentiment on social media or Slack channels at work using the defined meaning of the emojis or words that most people use, you will get it wrong for the subgroups that use them differently,” she said.
Evaluation bias
For bias arising when the benchmark data used for a particular task does not represent the population, the MIT paper offers facial recognition as an example, citing earlier work by Gebru and Joy Buolamwini. This work showed drastically worse performance of commercial facial analysis algorithms on images of dark-skinned women. That study noted that images of dark-skinned women comprise only 7.4% and 4.4% of common benchmark datasets.
Recommendations for keeping bias at bay in AI models
In the Salesforce guidelines, the authors enumerated several recommendations for enterprise to defend against bias and avoid traps lurking in datasets and the ML development process.
1. Verifiable data
Customers using an AI model as a service should be able to train the models on their own data, and organizations running AI should communicate when there is uncertainty about the veracity of the AI’s response and enable users to validate these responses.
The guidelines suggest that this can be done by citing sources, offering a lucid explanation of why the AI gave the responses it did — or giving areas to double-check — and creating guardrails that prevent some tasks from being fully automated.
2. Safety
Companies using AI should mitigate harmful output by conducting bias, explainability and robustness assessments, and red teaming, per the report. They should keep secure any personally identifying information in training data and create guardrails to prevent additional harm.
3. Honesty
When collecting data to train and evaluate models, organizations need to respect data provenance and ensure they have consent to use data.
“We must also be transparent that an AI has created content when it is autonomously delivered,” the report said.
4. Empowerment
AI developers should be cognizant of the distinction between AI projects ideal for automation, and those in which AI should be a subsidiary to a human agent.
“We need to identify the appropriate balance to ‘supercharge’ human capabilities and make these solutions accessible to all,” the authors wrote.
5. Sustainability
The guidelines suggest that users of AI should consider size and consumption of an AI model as part of their work on making them accurate to reduce the carbon footprint of these frameworks.
“When it comes to AI models, larger doesn’t always mean better: In some instances, smaller, better-trained models outperform larger, more sparsely trained models,” the MIT authors said.
Baxter agreed with that assessment.
“You have to take a holistic look when thinking about creating AI responsibly from the beginning of creating AI,” said Baxter. “What are the biases coming with your idea, along with the assumptions you are making, all the way through training, development evaluation, fine tuning and who you are implementing it upon? Do you give the right kind of remediation when you get it wrong?”