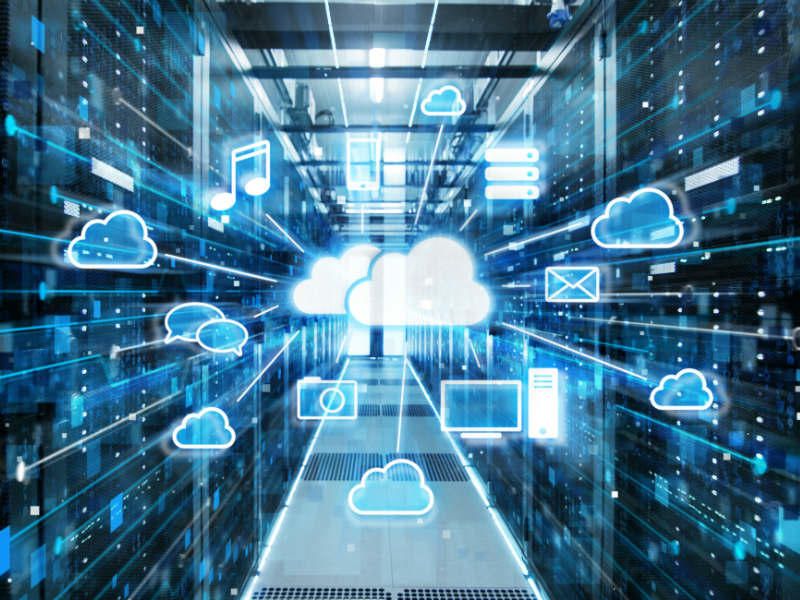
Duckbill Group’s chief cloud economist Corey Quinn knows a thing or two about shaving costs off your AWS bill, so when he suggests that keeping workloads in your data center might be a good idea, it’s worth paying attention. Specifically, Quinn queried if there’s a compelling “business case for moving steady-state GPU workloads off of on-prem servers,” because GPU costs in the cloud are incredibly expensive. How expensive? By one company’s estimate, running 2,500 T4 GPUs on their own infrastructure would cost $150K per year. On AWS running 1,000 of those same GPUs would cost … over $8M.
SEE: Hiring Kit: Cloud Engineer (TechRepublic Premium)
Why would anyone do that? As it turns out, there are very good reasons, and there are industries that depend on low-latency GPU-powered workloads. But there are also great reasons to keep those GPUs humming on-premises.
GPUs in the cloud
To answer Quinn’s question, it’s worth remembering the differences between CPUs and GPUs. As Intel details, though CPUs and GPUs have a lot in common, they differ architecturally and are used for different purposes. CPUs are designed to handle a wide variety of tasks quickly, but are limited in how they handle concurrency. GPUs, by contrast, started as specialized ASICs for accelerating 3D rendering. The GPU’s fixed-function engines have broadened their appeal and applicability over time but, to Quinn’s point, is the cost of running them in the cloud simply too high?
SEE: Guardrail failure: Companies are losing revenue and customers due to AI bias (TechRepublic)
That’s not the primary point, Caylent’s Randall Hunt responded. “Latency is the only argument there–if cloud can get the servers closer to the place they need to be, that can be a win.” In other words, on-premises, however much cheaper it may be to run fleets of GPUs, can’t deliver the performance needed for a great customer experience in some areas.
Like?
Well, how about video transcoding of live events, noted Lily Cohen? Sure, you may be able to get by with CPU transcoding with 1080p-quality feeds, but 4K? Nope. “Every second of delay is a second longer for the end user to see the feed.” That doesn’t work for live TV.
Nor is it just live TV encoding. “Basically anything that needs sub 100ms round trip” has latency demands that will push you to cloud GPUs, Hunt argued. This would include real-time game engines. “Streaming of real time game engines to do remote game development or any 3D development in them where accuracy matters” is cause for running GPUs close to the user, Molly Sheets stressed. For example, she continued, “‘[M]issing the jump’ when I’m runtime” ends up pushing you into “territory where you don’t know if it’s a Codec and how it renders or the stream.” Not a great customer experience.
If it sounds like GPUs are just here to entertain us, that’s not the case. “Any ML training workload that requires access to a large amount of data will need low latency, high throughput access to those data,” Todd Underwood suggested. (Not everyone agrees.) Add to that speech processing, self-driving cars, etc. Oh, and “renting” GPUs in the cloud can be the right answer for a wider variety of workloads if you simply can’t purchase GPUs to run locally in your own data center, given how demand can often exceed supply. Plus even if you can find them, your team may lack the capabilities to cluster them, something that Samantha Whitmore called out.
Which means that the ultimate answer to “should you run GPUs in the cloud” is sometimes going to be “yes” (when latency matters) and often going to be “it depends.” You know, the usual answer to computing questions.
Disclosure: I work for MongoDB but the views expressed herein are mine alone.